August 4-6, 2022
University of Washington, Seattle, WA
A number of domain applications of data science, machine learning, mathematical optimization, and control have underscored the importance of the assumptions on the data generating mechanisms, changes, and biases. Distributional robustness has emerged as one promising framework to address some of these challenges. This topical workshop under the auspices of the Institute for Foundations of Data Science, an NSF TRIPODS institute, will survey the mathematical, statistical, and algorithmic foundations as well as recent advances at the frontiers of this research area. The workshop features invited talks as well as shorter talk by junior researchers and a social event to foster further discussions.
Central themes of the workshop include:
- Risk measures and distributional robustness for decision making
- Distributional shifts in real-world domain applications
- Optimization algorithms for distributionally robust machine learning
- Distributionally robust imitation learning and reinforcement learning
- Learning theoretic statistical guarantees for distributional robustness
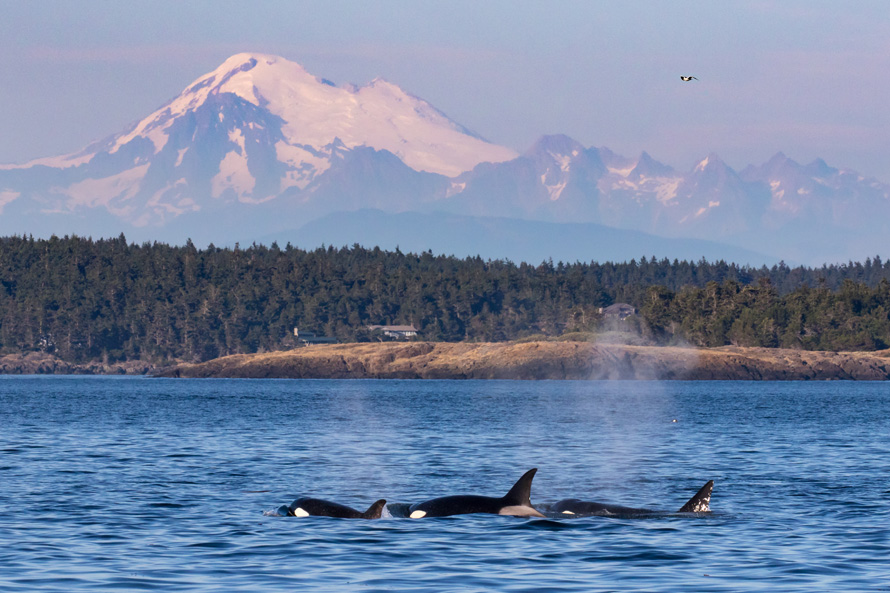